INSIGHTS

8 min read
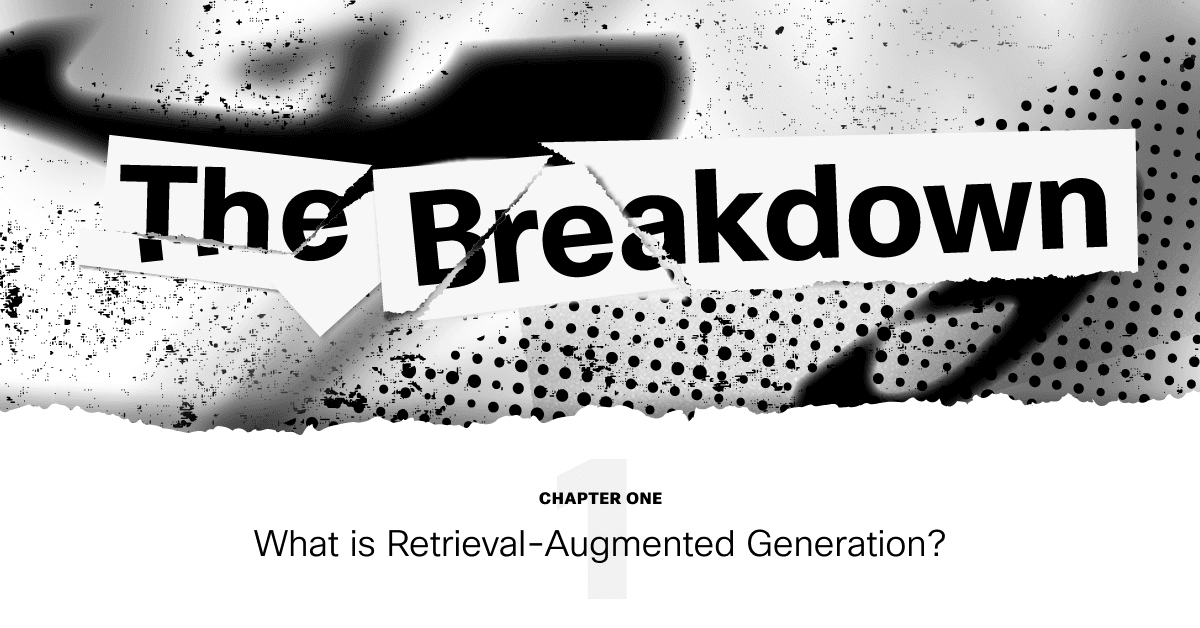
Published on 01/26/2024
Last updated on 03/04/2024
The Breakdown: What is retrieval-augmented generation?
Share
In the rapidly evolving field of AI, emergent tools like ChatGPT and Bard are bringing significant transformation to our technological landscape. These innovations embody a new era of AI that combines deep learning with highly nuanced, human-like interaction.
ChatGPT, a large language model (LLM) application developed by OpenAI, stands out for its ability to deliver responses that are not only contextually relevant, but also aligned with human values and expectations. As a result, it has become a tool with applications across multiple domains and industries. Businesses and professionals from every sector are keen to leverage the capabilities offered by ChatGPT and similar LLMs.
Embracing these advancements is not only advantageous but also indispensable for any organization aspiring to stay ahead in terms of innovation. Within the world of LLMs, one advancement gaining traction is retrieval-augmented generation (RAG), an approach that supplements the intelligent outputs of an LLM with just-in-time retrieval of up-to-date information.
In this article, we’ll break down RAG to give you a firm understanding of it. We’ll start by covering key concepts related to LLMs, and then we’ll dive deeper into RAG and how it comes into play. Finally, we’ll discuss how enterprises can leverage RAG alongside LLMs to provide accurate, relevant, and current results in their applications.
Key concepts to know
Let’s begin our breakdown of RAG with a primer on key terms.
Large language model (LLM)
An LLM is a type of generative AI (GenAI) algorithm trained on large datasets—typically petabytes in size—with complex learning techniques to understand, summarize, and generate content in natural human language.
Building an LLM is a complex process requiring tremendous computing resources. An LLM is a foundation on which an AI system can be built. Users can query the LLM with a prompt.
Training cutoff date
For LLMs like GPT, the training cutoff date refers to the latest point in time at which the model was trained with new data. For example, GPT-4 Turbo (version gpt-4-1106-preview) has a training cutoff date of April 2023. This means an LLM is at risk for generating inaccurate responses based on outdated data. This limitation becomes significant in continuously evolving domains like technology, world news, and current affairs.
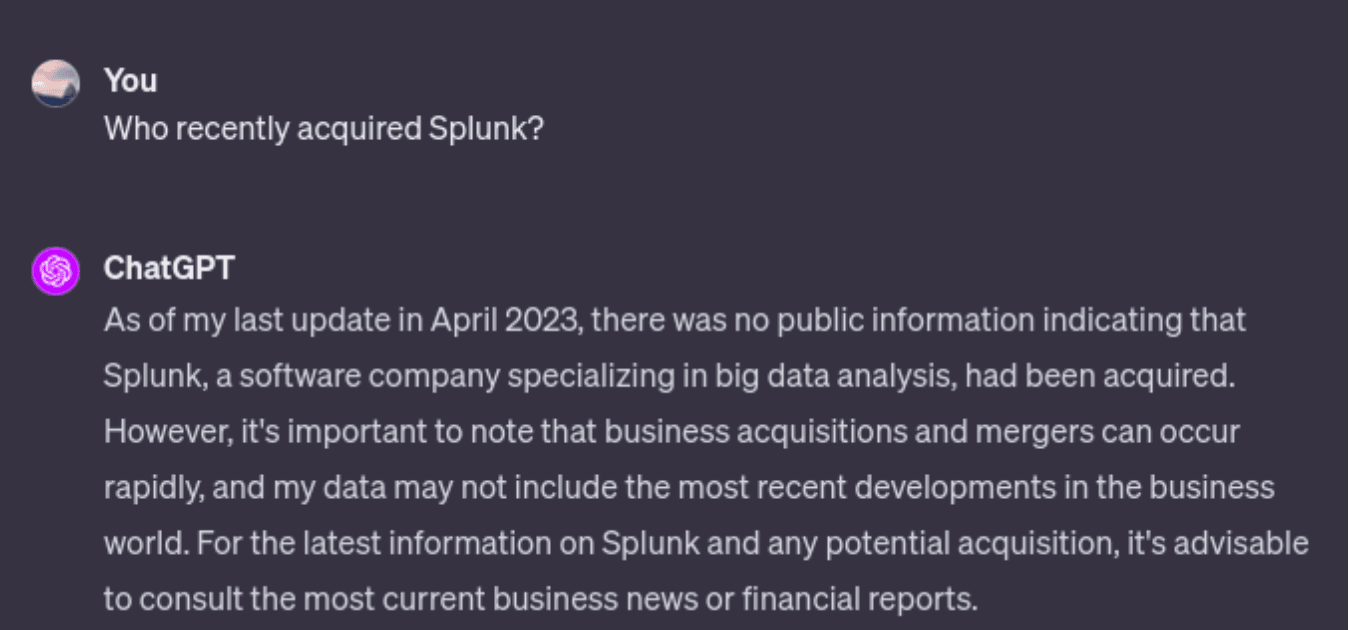
GenAI hallucination
A GenAI hallucination occurs when a GenAI model—in an effort to fulfill a request for content— produces information or a response that is inaccurate, nonsensical, or even completely unrelated to the user’s input. This can happen when a model encounters a question that is outside of its training scope.
Prompt engineering
Prompt engineering is the practice of crafting and designing specific instructions to guide an LLM to generate specific outputs. This might include providing additional context (which the LLM didn’t have when it was trained) or specifying response requirements such as length or format.
The limitations of LLMs
An LLM like GPT-4 has been trained on a massive amount of data. At first glance, one might think that the LLM knows practically everything there is to know. Yes, for most questions you might ask, LLMs like GPT-4 perform quite well—with two exceptions. An LLM cannot accurately answer questions about the following:
- Current information or news that occurred after the training cutoff date. For example, with its training cutoff date of April 2023, GPT-4 would be unable to tell you who won the 2023 FIFA Women’s World Cup, which took place later in the year.
- Private or proprietary information that was not part of the training dataset. For example, because GPT-4 didn’t train on your company’s internal records, it would be unable to answer questions about 2019 sales numbers from your Singapore office. Or, it can’t provide you with health guidance regarding your recent medical diagnosis, since it was not trained on your medical reports.
Without RAG, enterprises that want an LLM to answer these kinds of questions have one of two options:
- Build an LLM from scratch, training it with data that includes up-to-date information and the enterprise’s proprietary information.
- Fine-tune an existing LLM with up-to-date information and proprietary information.
Both of the above options are resource- and time-intensive. Many companies are unable to take either of these approaches. In addition, data privacy concerns would prevent companies from training an LLM on private or proprietary data.
Now that we have the key concepts laid out, let’s break down what RAG is and how it works.
Breaking it down
Retrieval-augmented generation (RAG) is an innovative technique that significantly enhances an LLM’s capabilities. When a user asks a question, RAG adds contextual information that can be applied to the LLM to address its knowledge limitations.
The main goal of RAG is to expand the knowledge base of an LLM by using external sources to improve the precision and dependability of LLM results. RAG allows LLMs to temporarily access and integrate data that it wasn’t trained on, thereby addressing their real-time knowledge limitations. Let’s dive in more deeply to break down how RAG works.
How RAG works
RAG works by supplementing the user’s original question with external information, and sending this all to the LLM when requesting a response. In essence, RAG is a unique way of doing prompt engineering, essentially saying:
- Start with all the information that you have been trained on.
- Take into account this new information that I’m providing to you.
- Now, based on all of this together, please answer my question.
One writer refers to RAG as the open-book test for generative AI:
RAG fills in the gaps in knowledge that the LLM wasn't trained on, essentially turning the question-answering task into an “open-book quiz.”
Or to use another analogy, we can think of RAG like a world-class chef preparing a meal. The chef (the LLM) has a vast array of ingredients (knowledge from training data). However, sometimes a diner (the user) asks for a dish with a specific, fresh ingredient that the chef doesn’t have in the kitchen.
Here’s where RAG comes in. RAG is like a chef’s assistant who runs out to the market (external data sources) to fetch the fresh ingredient (up-to-date or proprietary/sensitive information). The chef then combines this retrieved ingredient with their existing ones to create a dish that satisfies the customer’s request.
RAG has enhanced the traditional question-answer flow between a user and an LLM by introducing a retriever. When the user poses a question to the LLM, the retriever locates the relevant data and engineers a prompt for the LLM that combines this contextual information with the user’s query. With this information supplementing what it already knows, the LLM generates an answer that is both well-informed and up-to-date.
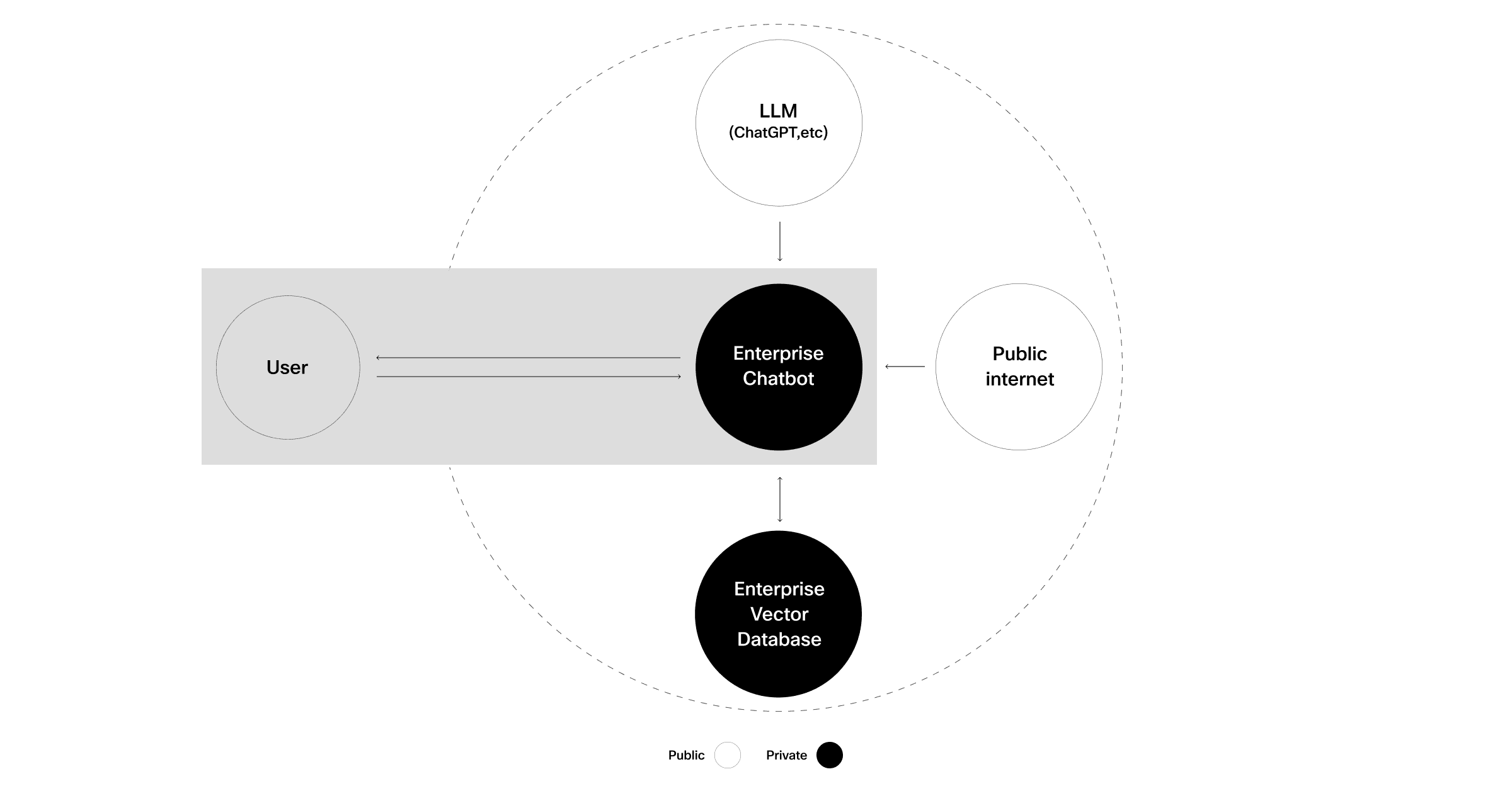
A RAG approach enhances LLM usage by supplying private or up-to-date information with precision and specificity. LLMs enhanced by RAG excel at addressing inquiries and conducting fact-finding tasks due to their access to supplemental information.
To summarize, RAG enhances GenAI capabilities by helping an LLM access and utilize information outside of its training dataset, resulting in accurate and relevant responses while reducing the risk of hallucinations. By supplementing LLMs with data on demand, the RAG approach does not require time-consuming or resource-intensive retraining or fine-tuning of an LLM, nor does it require giving up control of private information by including it in training data.
Use cases for an enterprise
By blending knowledge with external data, RAG represents a significant leap in how enterprises can leverage LLMs. Examples of RAG use cases for the enterprise include:
- Customer service: Promptly address customer inquiries by supplementing a pre-built LLM with company-specific product details and policies.
- Market analysis: Provide valuable insights into present market conditions by accessing up-to-the-minute data and news.
- Healthcare: Offer medical guidance based on the latest research and guidelines.
- Business operations: Assist employees with intelligent search of an organization’s internal documents and data.
RAG boosts the functionality of LLMs
LLM-based applications such as ChatGPT and Bard utilize datasets and sophisticated learning techniques to comprehend and generate content that closely resembles natural human language. However, these models are limited in what they “know” because they rely on fixed training data. Prompt engineering is a practice that involves designing prompts to achieve specific and efficient outcomes. This practice enhances the versatility of models across domains, ensuring their effectiveness.
RAG enhances LLMs by retrieving and integrating real-time information as part of the prompt engineering process. It overcomes the constraints imposed by fixed training data, reducing the need for LLM retraining or fine-tuning.
The current landscape in GenAI applications and innovation is evolving rapidly. Enterprises seeking to stay on the cutting edge of these developments must keep current in their understanding of new concepts and approaches, such as RAG. Outshift is at the forefront of equipping modern enterprises with the expertise and guidance they need to adopt AI in a manner that is not only effective but also responsible and trustworthy. For more information about Outshift’s role in the global discussion, read more on why we care about trustworthy and responsible AI.
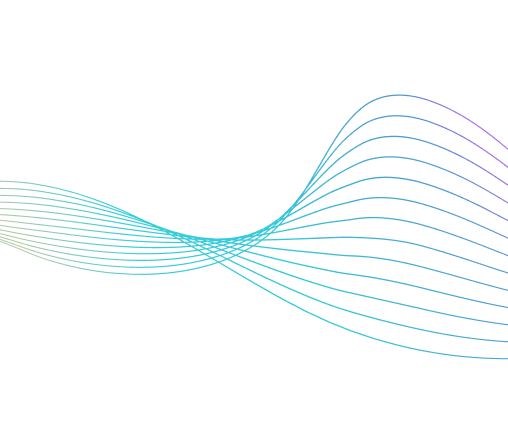
Get emerging insights on emerging technology straight to your inbox.
Unlocking Multi-Cloud Security: Panoptica's Graph-Based Approach
Discover why security teams rely on Panoptica's graph-based technology to navigate and prioritize risks across multi-cloud landscapes, enhancing accuracy and resilience in safeguarding diverse ecosystems.
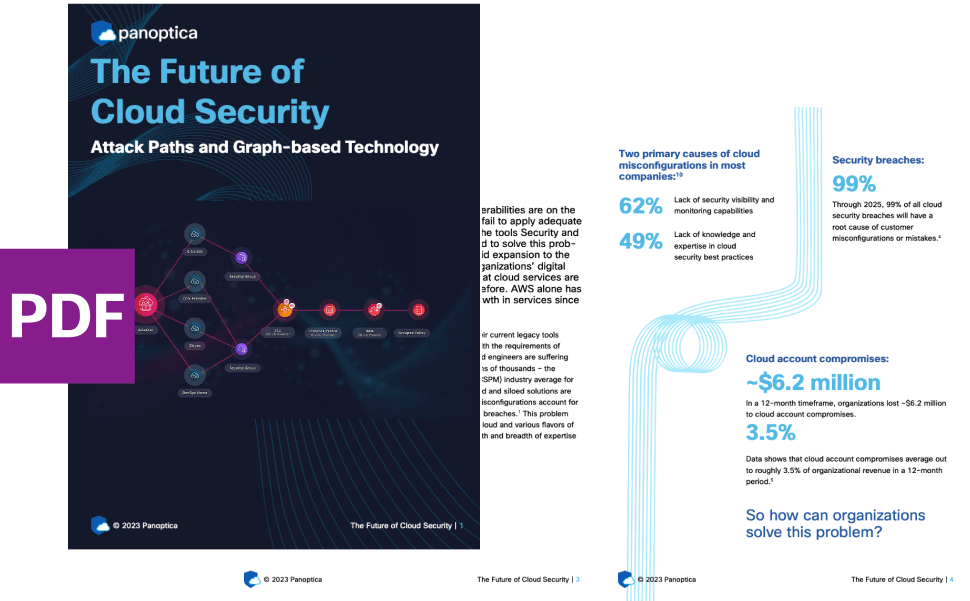
Related articles
The Shift keeps you at the forefront of cloud native modern applications, application security, generative AI, quantum computing, and other groundbreaking innovations that are shaping the future of technology.
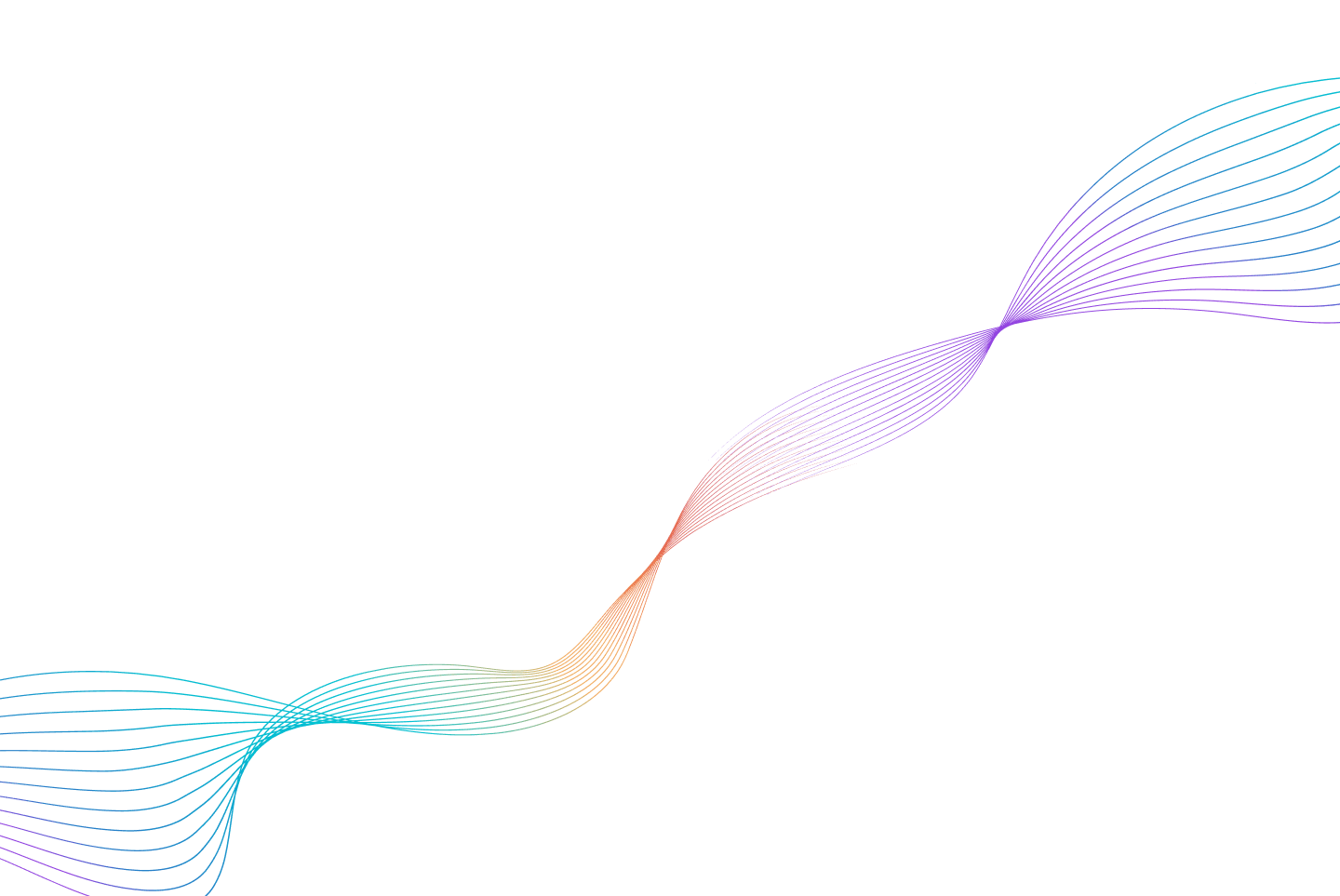